BETVLCTOR伟德官方网站2019级硕士研究生牛润良的论文AttExplainer: Explain Transformer via Attention by Reinforcement Learning近日被CCF-A类会议IJCAI-ECAI 2022接收。国际人工智能联合会议(International Joint Conference on Artificial Intelligence, 简称为IJCAI)是人工智能领域中最主要的学术会议之一。今年的第31届国际人工智能联合会议与第25届欧洲人工智能会议(European Conference on Artificial Intelligence,简称ECAI)联合举办。会议将于2022年7月23日至29日在奥地利首都维也纳进行。牛润良目前从事自然语言处理的研究工作,本篇文章与魏哲培同学、王岩老师、王琪老师合作完成。
论文题目:AttExplainer: Explain Transformer via Attention by Reinforcement Learning
第一作者:牛润良
第二作者:魏哲培
合作导师:王岩、王琪
会议详情:IJCAI-ECAI 2022, the 31st International Joint Conference on Artificial Intelligence and the 25th European Conference on Artificial Intelligence.
论文概述:
近年来Transformer模型及其各种变体为许多自然语言处理任务带来了显著的效果提升。但是Transformer模型结构复杂,堆叠层数多,这为模型解释带来挑战,也给使用过程中带来了潜在的风险。本文提出了一种基于强化学习的Transformer模型解释方法——AttExplainer。该方法构建了一个强化学习环境,在每一时间步,智能体代理观测注意力矩阵并选择一个字符进行掩码操作。持续这样的操作,直到Transformer模型的分类决策发生改变或分类概率大幅度下降。最终找到的被掩码的部分是支撑原本分类决策的关键字符。本文在三个常用文本分类数据集上进行了实验,在模型解释和模型对抗攻击这两个任务中取得了比基线方法更好的成绩。通过对比实验证明了注意力矩阵中确实含有潜在的关于字符重要性的信息,为注意力矩阵的可解释性提供证据支持。同时发现这种潜在的可解释性可以在不同的数据集和已训练模型之间迁移。
Transformer and its variants have recently achieved remarkable performance in many NLP tasks. However, the complex structure of Transformer and the huge number of stacked layers pose challenges for model interpretation and potential risks in their use. In this paper, we propose a novel reinforcement learning (RL) based framework for Transformer explanation via attention matrix, namely AttExplainer. The method builds a reinforcement learning environment. In each time step, the RL agent learns to perform step-by-step masking operations by observing the change in attention matrices, until the classification decision of Transformer changes or the probability decreases significantly. Then it can be shown that the masked part contains key positions that support the original classification decision. We have adapted our method to two scenarios: perturbation-based model explanation and text adversarial attack. Experiments on three widely used text classification benchmarks validate the effectiveness of the proposed method compared to state-of-the-art baselines. Additional studies show that our method is highly transferable.
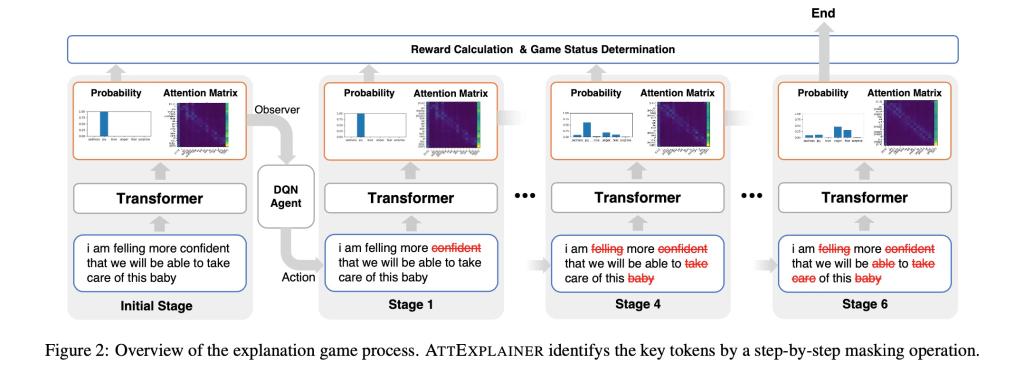