报告题目: Towards Trustworthy Learning and Reasoning under Noisy Data
报告题目:噪声数据下的可信学习与推理
报告摘要:
Trustworthy learning and reasoning are the emerging and critical topics in modern machine learning, since most real-world data are easily noisy, such as online transactions, healthcare, cyber-security, and robotics. Intuitively, trustworthy intelligent system should behave more human-like, which can learn and reason from noisy data. Therefore, in this talk, I will introduce trustworthy learning and reasoning from three human-inspired views, including reliability, robustness, and interaction. Specifically, reliability will consider uncertain cases, namely deep learning with noisy labels. Meanwhile, robustness will discuss adversarial conditions, namely deep learning with noisy (adversarial) features. Then, interaction will focus on the dynamic interaction between noisy labels and noisy features. Besides labels and features, I will discuss other noisy data, such as noisy domains, noisy demonstrations, and noisy graphs. Furthermore, I will introduce the newly established Trustworthy Machine Learning and Reasoning (TMLR) Group at Hong Kong SAR and Greater Bay Area.
报告摘要:
由于医疗保健、在线交易、网络安全和机器人等应用中的数据通常含有噪声,可信学习与推理成为了数据驱动的现代机器学习领域的新兴关键主题。可靠的智能系统应该像人类一样,能够从噪声数据中学习和推理。在本次报告中,将从可靠性、鲁棒性和交互性三个受人类启发的观点出发,介绍可信学习与推理。具体的,可靠性研究考虑的是存在噪声标签的不确定场景下的深度学习,鲁棒性研究讨论的是存在噪声(对抗)特征的对抗环境下的深度学习,交互性研究关注的是噪声标签与噪声特征之间的动态交互。除了特征与标签外,将讨论其他噪声数据类型,例如噪声域、噪声演示和噪声图。最后,将介绍在香港特别行政区和大湾区新成立的可信机器学习与推理 (Trustworthy Machine Learning and Reasoning,TMLR) 课题组。
报告人简介:
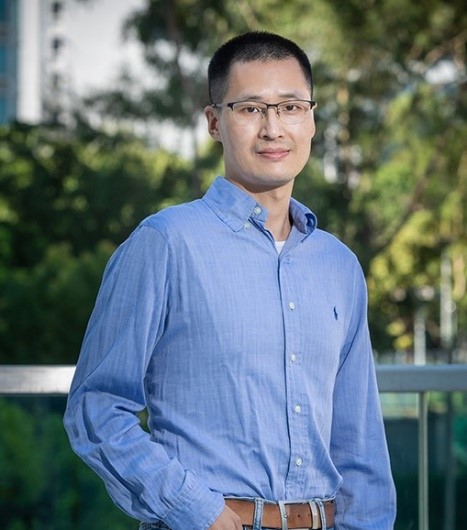
韩波,香港浸会大学计算机系助理教授,领导TMLR研究组。他同时兼任RIKEN人工智能项目梅峰访问科学家,并曾兼任Microsoft研究院访问科学家。他于2019-2020年博士后于RIKEN人工智能项目;2019年博士毕业于悉尼科技大学。他的研究广泛涉猎了机器学习的诸多理论和实践领域,目前关注重点包括可信赖表示学习,因果表示学习等方法,以及这些方法在自然科学和交叉学科的应用。他的研究得到众多政府和业界资金支持,并获得众多奖项,包括三项政府研究奖 (RGC CAREER, NSFC Young Scientist, RIKEN BAIHO),和五项企业研究奖 (Microsoft, Nvidia, Huawei, Tencent, Alibaba)。
报告时间:2022年10月18日 星期二 13:30-15:00
报告地点:腾讯会议:458-698-826